React and D3 Integration
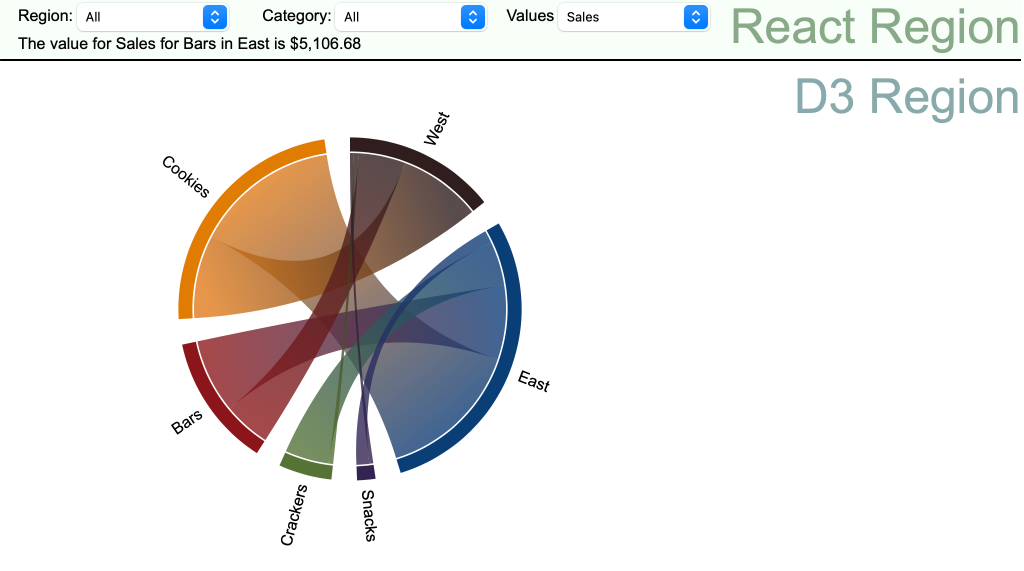
There is a YouTube video for this demo here: https://youtu.be/XdPBM5N07cE
This shows how to use the visualization library D3 with the React framework. This not trivial because D3 works by directly manipulating an SVG object with the HTML DOM, whereas React works with a virtual DOM that is converted and injected into the HTML page at render time. The video has a complete walkthrough and this post explains some of the details in the video.
If you are new to React these are the steps I took to create the project. You will need to have the React and NPM installed already. You might need to use ‘sudo’ for the npx and chown commands depending on your security configuration. These commands are what I used on my Mac but React will install on Windows and Linux as well. The installation and setup are not the purpose of this BLOG post, I’m including them so you can set up the demo to work on your system. You can skip all of these steps if you just want to read about how to do the integration.
Setup
Create the React project:
npx create-react-app d3-demo
Change the ownership if you need to:
sudo chown -R {your user name} d3-demo
Run the default project to make sure basic setup is ok:
cd d3-demo
npm start
Your browser should open at http://localhost:3000 and show you the default React page. You can download all the demo files here. It’s just the Javascript and React source files to drop into you react directory structure.
https://www.dropbox.com/s/lgzms6283r31d6l/React-D3.zip?dl=1
The directory structure should look like this
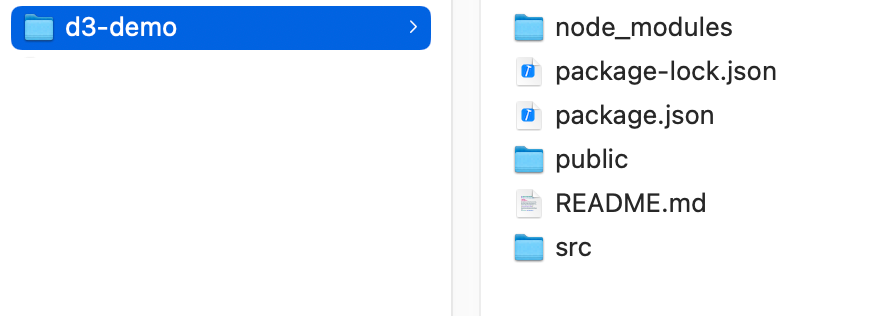
D3/React Integration
First public/index.html. It’s quite simple.
<!DOCTYPE html>
<html lang="en">
<head>
<link rel="stylesheet" href="/Demo/Demo.css">
<!-- Make sure global.js is first. -->
<!-- The chart code and React code will add functions to its objects -->
<script src="/Demo/global.js"></script>
<!-- The D3 V3 library -->
<script src="/Demo/d3.v3.min.js"></script>
<!-- A D3 chord chart -->
<script src="/Demo/chart.js"></script>
<title>React/D3 Demo</title>
</head>
<body>
<!-- the React root. React "owns" this -->
<div id="React-Root"></div>
<div id="chart-label" class="ChartLabel">D3 Region</div>
<!-- the chart div where the D3 chart is created -->
<div id="D3-Chart" class="ChartDiv"></div>
</body>
</html>
The imports are global.js (see later), D3 V3, and chart.js (our chord chart, see later). You can see the “React-Root” div where the React components are injected into the HTML DOM.
You can also see the “D3-Chart” div where D3 adds its SVG object.
The Javascript file public/global.js is where the integration points are defined.
// These are React functions that can be called from Javascript
window.ReactFunctions = {};
// These are Javascript functions that can be called from React
window.JavascriptFunctions = {};
It defines two Javascript objects that the Javascript code and the React code use to provide functions that the other technology can call.
On line 11 in public/chart.js the function to replace the chord chart with a new one based on fresh data – updateChart is added to window.JavascriptFunctions. This can now be called from a React Component.
window.JavascriptFunctions.updateChart = function(d) {
...
}
Looking at the React code we can see in src/ValueHint.js (which provides the mouse-over hint) that on line 13 ValueHint makes the React function chartMouseOver available to Javascript code.
// make the mouseover function available to D3/Javascript
window.ReactFunctions.chartMouseOver = chartMouseOver;
Going back to public/chart.js we can see where this React function is called when the user moves the mouse over part of the D3 chord chart (line 150-164).
function pathMouseOver(d) {
if (d.source && d.target ) {
// Identify the information for the mouse over
var source = chartData.items[d.source.index];
var target = chartData.items[d.target.index];
var measure = chartData.value;
var value = chartData.matrix[d.source.index][d.target.index];
// Call the Reaction function to display the mouse over hint
console.log("Calling window.ReactFunctions.chartMouseOver "+target+" "+source);
window.ReactFunctions.chartMouseOver(source, target, measure, value);
}
} // pathMouseOver
The YouTube video linked above and the demo project files should help you understand what is happening on both the React and D3 side of the integration.
On “selling to developers” and “monetizing the community”
After a decade of open source business models, the leaders of open source companies are still making fundamental mistakes.
Today’s example is provided by Peter Goldmacher, VP of Strategy and Market Development at Aerospike. In a post by Dan Woods at Forbes titled “Why Open Source Does Not A Business Make” Golmacher talks about “selling to developers”.
This is the same mistake that VCs and executives make when they use the phrase “monetizing the community”. Your customers are not the developers, unless the developers pay for the software themselves and don’t expense it. Why? Because developers typically have no budget. The litmus test for this is the question “if the developer leaves their current employer and joins another, who is my customer now?” In almost all cases the answer is “their former employer”. A simpler question is “who signed the contract, a developer personally, or a representative of the company?” The developer may still be a part of the community, but the customer remains the company that wrote the check.
The correct way to think about this is to consider developers as a group who can sell the software for you. Looking at it this way has several advantages:
- You recognize that developers are not the final decision maker
- You realize that developers have one set of needs, and that corporations have additional or different needs
- You realize that you can provide tools and guidance to help developers sell your solution to management
As I state in the Beekeeper Model
Customers are corporations, the community are people. They have very different needs.
For more detail on this you can read this section of the Beekeeper Model.
It’s possible Goldmacher was paraphrased or miss-quoted about “selling to developers”. If he wasn’t this VP of Strategy might consider adjusting his strategy.
The Next Big Thing: Open Source & Big Data
These are the main points from the Open Source & Big Data section of my Next Big Thing In Big Data presentation.
- Open source fuels big data
- Big data fuels large enterprise
- Large enterprise fuels interest
- Interest fuels adoption and contribution
- Adoption and contribution benefits open source
- Open source has won
- Watch Apache projects for future standard building blocks
Please comment or ask questions
The Next Big Thing: Big Data Use Cases
These are the main points from Big Data Use Cases section of my Next Big Thing In Big Data presentation.
- Use cases are important for the big data community
- SQL on Big Data
- Data Lakes
- Real-Time/Streaming
- Big data should be designed in
- The use cases will change
Please comment or ask questions
The Next Big Thing: Tomorrow’s Technologies of Choice
These are the main points from Tomorrow’s Technologies of Choice section of my Next Big Thing In Big Data presentation.
- If data is more valuable than software, we should design the software to maximize the value of the data
- Data treatment should be designed-in
- Old architectures don’t work for new problems
- Scalable technology is better than big technology
Summary
- Data is valued
- New architectures
- Open source platforms
- Analysis is built in
Please comment or ask questions
The Next Big Thing: Today’s Technologies of Choice
These are the main points from Today’s Technologies of Choice section of my Next Big Thing In Big Data presentation.
- Interest in Hadoop ecosystem continues to grow
- Interest in Spark is growing rapidly
- R is important
- SQL on Big Data is a problem today
- Application developers leave data analysis for version 2
- Application architecture and big data architecture are not integrated or common
Summary
- Hadoop ecosystem is vibrant
- Analysis is an after-thought
Please comment or ask questions
The Next Big Thing: Data Science & Deep Learning
These are the main points from Data Science & Deep Learning section of my Next Big Thing In Big Data presentation.
- Value has drifted from hardware, to software, to data
- The more granular the data, the harder the patterns are to find
Summary
- Value of data as a whole is increasing
- The value of data elements is decreasing
- Data scientists don’t scale
- Tools are too technical
- Correct conclusions can be hard
- Something has to change
Please comment or ask questions
Next Big Thing: The Data Explosion
These are the main points from The Data Explosion section of my Next Big Thing In Big Data presentation.
- The data explosion started in the 1960s
- Hard drive sizes double every 2 years
- Data expands to fill the space available
- Most data types have a natural maximum granularity
- We are reaching the natural limit for color, images, sound and dates
- Business data also has a natural limit
- Organizations are jumping from transactional level data to the natural maximum in one implementation
- As an organization implements a big data system, the volume of its stored data “pops”
- Few companies are popping so far
- The explosion of hype exceeds the data explosion
- The server, memory, storage, and processor vendors do not show a data explosion happening
Summary
- The underlying trend is an explosion itself
- The explosion on the explosion is only minor so far
- Popcorn Effect – it will continue to grow
Please comment or ask questions
The Software Paradox by Stephen O’Grady
O’Reilly has released a free 60 page ebook by Stephen O’Grady called The Software Paradox – The Rise and Fall of the Software Industry. You can access it here.
So what is “The Software Paradox” according to O’Grady? The basic idea is that while software is becoming increasingly vital to businesses, software that used to generate billions in revenue is often now available as a free download. As the author says:
This is the Software Paradox: the most powerful disruptor we have ever seen and the creator of multibillion-dollar net new markets is being commercially de- valued, daily. Just as the technology industry was firmly convinced in 1981 that the money was in hardware, not software, the industry today is largely built on the assumption that the real revenue is in software. The evidence, however, suggests that software is less valuable—in the commercial sense—than many are aware, and becoming less so by the day. And that trend is, in all likelihood, not reversible. The question facing an entire industry, then, is what next?
The ebook is well researched, well thought-out, and worth a read if you work in the software industry.
O’Grady describes the software industry from its early beginnings to today, and considers the impact of open source, subscription models, and the cloud on many aspects of the industry. His conclusions and his summary are very interesting. However I think he as overlooked, or under-stated, two aspects.
The Drift to the Cloud
O’Grady identifies cloud computing as a disruptive element and provides details on the investments by IBM, Microsoft, and SAP in their cloud offerings, as well as the impact of the Amazon Cloud. He does a good job of describing who did what and when, but does not really get to the bottom of the “why”, other than pointing to consumer demand and disruption in the traditional sales cycle.
Here is my take on why the demand for hosted and cloud-based offerings is increasing and will continue to increase. Consider the evolution of a new business started in the last 5 years. As an example let’s use a fictitious grocery store called “Locally Grown” that cooperates with a farmer’s market to sell produce all week long instead of just one or two days a week.
- Locally Grown opens one store in San Diego. It uses 1 laptop and desktop-based software for all it’s software needs including accounting, marketing etc.
- Things go well and the owner opens a second Locally Grown across town. The manager of the second store emails spreadsheets of sales data so the accounting software can be kept up to date.
- When the third store opens, the manual process of syncing the data gets to be too much. The company switches to Google Docs and an online accounting system. This is an important step, because what they didn’t do was to buy a server, and hire an IT professional to setup on-premise systems, and configure the firewall and the user accounts etc.
- As the company grows a payroll system is needed. Since accounting and document management are already hosted, it is easiest to adopt a hosted payroll system (that probably already integrates with the accounting system).
- Soon an HR system is needed, and then a CRM system. As each system is added, it makes less and less sense to choose an on-premise solution.
You can see from this example that the important decision to choose hosted over on-premise is made early in the company’s growth. Additionally, that decision was not made by an IT professional, it was made by the business owner or line-of-business manager. Hosted application providers aim to make it easy to set up and easy to migrate from desktop solutions for this reason. By comparison the effort of setting up an on-premise solution seems complicated and expensive to a business owner.
I love me a good analogy, and Amazon’s Jeff Bezos has a good one for the software industry by comparing it to the early days of the electricity industry (TED talk here). I think the analogy goes further than he takes it. In the early days of the electricity grid, the businesses most likely to want to be connected were small ones without electricity, and those least needing the grid were large businesses that had their own power plants. This same effect can be seen with cloud adoption as small businesses with no server rooms or data centers are the most likely to use the cloud for all their needs, and large businesses will be the slowest to migrate.
In their Q1 2016 annual report Salesforce announced $1.41 billion in subscription and support revenue. They don’t release information about their customers or subscribers but it is generally know they have over 100,000 customers. But consider that in the USA there are 28 million small businesses (less than 500 employees). These are the next generation of medium sized, and then large businesses. Even if we are generous and put Salesforce’s customer count at 200,000 in the USA (by ignoring the other 20 countries), it means that Salesforce has a market penetration of less than 1% and still generates $6bn a year. So just within the hosted CRM space in the USA alone the market is more than $600bn a year.
So, in my opinion, the current demand for hosted and cloud-based offerings is largely (or at least significantly) fueled by the latest generation of small businesses that will never have an on-premise solution. This trend will continue relentlessly, and perpetually (until something easier and cheaper emerges), and the market is vast.
New License Revenue
O’Grady does a lot of analysis of software license revenues over the past 30 years and compares it with subscription models and open source approaches. But he is missing a fact that might alter his opinions a little. Most people are unaware of this fact, because traditional software companies have a dirty little secret.
It is rational to think that when you buy a piece of software from IBM, or Oracle, or SAP, or Microsoft, that the money you give them pays for your part of the development of the software, the cost of delivering it, the cost of running the business, and a little profit on top. But this is unfortunately not the case. When you buy a new software license the fee you pay typically covers only the sales and marketing expenses. In extreme cases it doesn’t even cover those. In the past, Oracle’s sales and marketing departments were allowed to spend up to 115% of the new license revenue. Oracle was losing money just to acquire customers. In the latest Oracle quarterly report they state that new license revenue was $1,982 million and sales and marketing expenses was $1,839 million. So 92% of your license fee goes towards the sales and marketing expenses needed to get you to buy the software, and the remaining 8% doesn’t even come close to covering the rest. As a consequence, a traditional software company is not at all satisfied that you have just purchased software from them because they have made a loss on the deal.
So if the license fee does not pay for the software, what does? It is the renewals, upgrades, up-sells, support, and services that generate the income that funds the development of the software and keeps the lights on.
In The Software Paradox O’Grady talks about the massive rise in software revenue license in the 1980s and 1990s, and it’s subsequent decline. But if you consider that software license revenue is really just fuel for sales and marketing, maybe the decline can be partially attributed to innovation in the sales and marketing worlds (evaluation downloads, online marketing etc) as the heavy and expensive enterprise sales model has changed over time. Maybe the 1980s and 1990s was a sellers market and expensive software of the time was overpriced. O’Grady describes many contributing factors for the Software Paradox, maybe this is yet one more.
Pentaho Labs Apache Spark Integration
Today at Pentaho we announced our first official support for Spark . The integration we are launching enables Spark jobs to be orchestrated using Pentaho Data Integration so that Spark can be coordinated with the rest of your data architecture. The original prototypes for number of different Spark integrations were done in Pentaho Labs, where we view Spark as an evolving technology. The fact that Spark is an evolving technology is important.
Let’s look at a different evolving technology that might be more familiar – Hadoop. Yahoo created Hadoop for a single purpose indexing the Internet. In order to accomplish this goal, it needed a distributed file system (HDFS) and a parallel execution engine to create the index (MapReduce). The concept of MapReduce was taken from Google’s white paper titled MapReduce: Simplified Data Processing on Large Clusters. In that paper, Dean and Ghemawat describe examples of problems that can be easily expressed as MapReduce computations. If you look at the examples, they are all very similar in nature. Examples include finding lines of text that contain a word, counting the numbers of URLs in documents, listing the names of documents that contain URLs to other documents and sorting words found in documents.
When Yahoo released Hadoop into open source, it became very popular because the idea of an open source platform that used a scale-out model with a built-in processing engine was very appealing. People implemented all kinds of innovative solutions using MapReduce, including tasks such as facial recognition in streaming video. They did this using MapReduce despite the fact that it was not designed for this task, and that forcing tasks into the MapReduce format was clunky and inefficient. The fact that people attempted these implementations is a testament to the attractiveness of the Hadoop platform as a whole in spite of the limited flexibility of MapReduce.
What has happened to Hadoop since those days? A number of important things, like security have been added, showing that Hadoop has evolved to meet the needs of large enterprises. A SQL engine, Hive, was added so that Hadoop could act as a database platform. A No-SQL engine, HBase, was added. In Hadoop 2, Yarn was added. Yarn allows other processing engines to execute on the same footing as MapReduce. Yarn is a reaction to the sentiment that “Hadoop overall is great, but MapReduce was not designed for my use case.” Each of these new additions (security, Hive, HBase, Yarn, etc.) is at a different level of maturity and has gone through its own evolution.
As we can see, Hadoop has come a long way since it was created for a specific purpose. Spark is evolving in a similar way. Spark was created as a scalable in-memory solution for a data scientist. Note, a single data scientist. One. Since then Spark has acquired the ability to answer SQL queries, added some support for multi-user/concurrency, and the ability to run computations against streaming data using micro-batches. So Spark is evolving in a similar way to Hadoop’s history over the last 10 years. The worlds of Hadoop and Spark also overlap in other ways. Spark itself has no storage layer. It makes sense to be able to run Spark inside of Yarn so that HDFS can be used to store the data, and Spark can be used as the processing engine on the data nodes using Yarn. This is an option that has been available since late 2012.
In Pentaho Labs, we continually evaluate both new technologies and evolving technologies to assess their suitability for enterprise-grade data transformation and analytics. We have created prototypes demonstrating our analysis capabilities using Spark SQL as a data source and running Pentaho Data Integration transformations inside the Spark engine in streaming and non-streaming modes. Our announcement today is the productization of one Spark use case, and we will continue to evaluate and release more support as Spark continues to grow and evolve.
Pentaho Data Integration for Apache Spark will be GA in June 2015. You can learn more about Spark innovation in Pentaho Labs here: www.pentaho.com/labs.
I will also lead a webinar on Apache Spark on Tuesday June 2, 2015 at 10am/pt (1pm/ET). You can register at http://events.pentaho.com/pentaho-labs-apache-spark-registration.html.